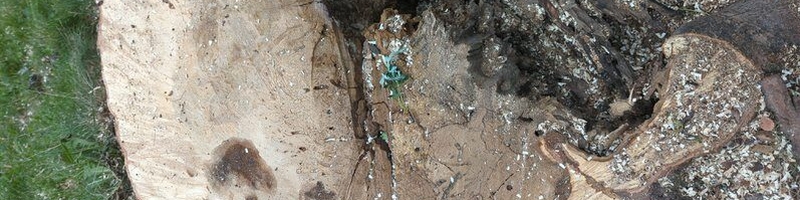
Orbiting Jupiter
During my undergrad, I spent a lot of my free time trying to write a novel. At the time, the project meant a lot to me, but it was a doomed endeavour from the start; I’m just not good at writing characters. The novel was less a coherent story and a more random collection of scenes that I desperately tried to stitch together into a coherent narrative. One of the scenes took place on board a space station orbiting Jupiter. Humanity had progressed to the point where we were spread out across the solar system, but had not yet learned to exist meaningfully in space. Jupiter was an important source of minerals for the wider human civilization, but was otherwise a miserable place to be.
To cope, the residence of Jupiter Station had voluntarily enclosed themselves in a self-created meta-verse. A virtual existence, wholly separate from the physical realities of living on a space station; like the Matrix, only not a secret. This meta-verse existed more like Star Trek’s Holodeck than the simulacrum of real life portrayed in the movie. The residence of Jupiter Station needed only to image something, and they would have it: Sex in as much abundance and variety as they wished, drugs of any potency without any side effects, food of any variety and in any quantity, and most importantly power over anything they desired. The fantasy they could create for themselves would be as unique or mundane as they wanted it to be; a paradise of human desire, free of any troubles or issues that may come up in day to day living. A reasonable surface level end point if we assume infinite technological progression.
What are the consequences of a world devoid of nature, yet optimized for human value?
The story includes a religious group who reject the fantasy that is presented to them. They view the meta-verse as a great evil and work to undermine it. Unfortunately, they are successful and manage to permanently disable the computers that generate the illusions, forcing the residence to face reality, and causing the society to collapse. Some residences killed themselves unable to part with the loss of their personal universe, others, who had the means, left. Those left behind all died trapped inside an artificial metal monstrosity without the means, knowledge, or even ability to sustain themselves. Even the religious organization that wanted this world died because they too vastly underestimated their own reliance on the very system they hated.
As a novel it never panned out, but as a philosophical experiment it lives on in my head. If technology continues to advance, and we keep solving problems to make life better for ourselves, why is the result so fragile? What are the consequences of a world devoid of nature, yet optimized for human value?
Optimization
The first and most important lesson one must learn when dealing with any applied mathematics is that it is impossible to optimize two variables at the same time. A good example of this is traffic.
Say we want to minimize a car’s travel distance between two points. On the surface, this seems like an easy problem; we increase the speed limit, pave the road as straight as possible, and finish our job under budget. However, things change when we add a second car to the road. No longer can we just let them both drive as fast as possible down a straight highway as these two cars may interact with each other, and we need to account for this possibility. The faster these cars are going, the more catastrophic it will be if they collide, preventing either from reaching their destination and blocking further traffic until the debris is cleared. We could prevent such an accident by imposing speed limits, thereby limiting the severity of the crash, but doing so would conflict with our initial goal of reducing travel time.
In reality, any real road project is trying to optimize way more variables than just two cars: budget, land use,
environmental impact, impact on neighbouring landowners, and of course the lives of the thousands if not millions of
people who will be using it every day. All of these variables are important, and no solution can universally
optimize on all of
them at once; every decision has a cost. Some costs are explicit, like the road’s price tag. Some are understood but
accepted, such as the resulting noise and air pollution caused by traffic. Others are external and not allowed into
the accounting to begin with, such as the impact on the area’s wildlife. We can work around some of these issues by
coming up with mathematical or social models that convert some variables into others. Instead of dealing with
individual drive times, we can work with statistical measures: How can we ‘minimize’ the ‘average’ travel time on a
road for all users? What is the ‘longest trip’ someone will have to make? How can we reduce the ‘probability’ that a
collision will occur? Likewise, we can pick social models to reduce the variables. We can optimize for ‘safety’ by
slowing everyone down to reduce the risk of accident, or we can optimize for ‘choice’ by creating different
lanes with different rules and allow users to choose their level of risk. Of course, all of these models make some
assumptions about what we humans value and are nothing more than statistical tricks to reduce the problem to a
single measurable variable.
Once we have selected our objective, or target as it is commonly referred, the act of optimization itself can be thought of as a game1. The singular variable we are optimizing on is the game’s goal, and all the variables we can manipulate are its structure. Regardless of how many players are playing the game, the winner is the person who develops the best strategy to optimize the desired target. Alan Turing2 used such a game to argue that computers can think, and at the same time created the framework of target generation that all modern artificial intelligence systems employ. He called this game the Imitation Game.
The Imitation Game
Imagine a game with three players: one human, one computer, and the third an interrogator with no knowledge of the other two. Both the human and the computer are trying to convince the interrogator that they are the human, and the interrogator is tasked with determining who is telling the truth. The optimal strategy for the computer (which Turing referred to as A) is to impersonate a human as good as possible, while the human (which Turing referred to as B) is trying to reveal this deception. Turing’s goal in introducing this game was to reduce a complicated question like, “can computers think” into a single model we could then theorize about: can the computer win?
We now ask, “What will happen when a machine takes the part of A in this game?” Will the interrogator decide wrongly as often when the game is played like this…? These questions replace our original, “Can machines think?”
Alan Turing, Computing Machinery and Intelligence (pp 50)3
To Turing, the question of “can machines think?” was ambiguous because ‘thinking’ had no non-subjective definition. What it meant to ‘think’ was, and remains, a very philosophical concept that is warped by whatever linguistic context it is used in. This objection is further reinforced in the second half of his paper, where he addresses the ‘argument from various disabilities.’ This argument is a generalized version of the claim that a ‘computer can never do X’, where X is any number of activities from ‘being friendly,’ to ‘enjoying strawberries,’ to ‘being the subject of its own thought.’ To Turing, this entire class of objections really boils down to the objection of consciousness. The objection John Searle brings up with his ‘Chinese room argument’ where he argues that a computer may be able to transform text perfectly, but that doesn’t mean it has an internal understanding or experience of its actions. Turing rejects this:
Likewise according to this view the only way to know that a man thinks is to be that particular man. It is in fact the solipsist point of view. It may be the most logical view to hold but it makes communication of ideas difficult. A is liable to believe “A thinks but B does not” whilst B believes “B thinks but A does not.” Instead of arguing continually over this point it is usual to have the polite convention that everyone thinks.
Alan Turing (pp 57)
The only reason we believe others think, is because they act in such a way that makes me believe they think. Thus, thinking is already an imitation game played between humans, and why should we exclude the non-human from playing it as well?
Yet this line of thinking is not without consequences. By arguing that imitation can replace the need for a definition, he is also arguing against the need for a definition at all. It is not necessary to understand how humans think or why humans think, it is only necessary to accept that thinking is a social construct that can be assigned to everything and anyone so long as they adhere to the social contract. A human is only what is perceived to be human, nothing else. In terms of optimization, Turing removes the need for a theoretical target completely. To reduce a multivariate problem to a single variable, we only need to double down on human intuition. In terms of our original problem, the best, most efficient road system is the one that humans like: the mathematical properties of such a system are irrelevant.
In terms of our original problem, the best, most efficient road system is the one that humans like: the mathematical properties of such a system are irrelevant.
Importantly, Turing didn’t introduce the imitation game using a computer, his opening paragraph introduces it as a game between a man and a woman. The man was trying to convince the interrogator that he was the woman. Turing moved away from this version of the game before the introduction to his lengthy paper had concluded, but its existence is, in my opinion, more important than the rest of the paper because it implies that the definition of the imitation game is not limited to computers, but is intended to be broadly applied. The imitation is available to be used to define anything that humans intuitively understand but is otherwise hard to define. It is the equivalent of Judge Potter Stewart’s, “I know it when I see it” when discussing what is and is not pornography: except applied to everything. What is justice if not something that is perceived as being just? What is ethics if not something that is perceived as being ethical? What is a woman if not something that is perceived as being a woman? What is truth if not something that is convincing. The imitation game is a rejection of philosophy, an admittance that the Greek sophists were right. It’s not important that something exists objectively, it is only important that it acts convincingly.
It is a meta-verse. A universe of our own creation, and the imitation game gives computer scientists a way to drag this fantasy into their mathematical models.
Machine Learning
Computer intelligence is simultaneously the easiest thing on the planet to explain, and so difficult that even those who study it have no idea how it works. At its core, the entire field of AI is a very complicated imitation game. To create an AI, we begin with a ‘Data Scientist’ who decides how the question ‘what is convincing’ can be programmed into a computer. Usually this is done by asking billions of humans questions like “Does this picture look like a bird?” then storing the answers in enormous datasets4. The actual training is itself a game computers play against themselves. It begins when the Data Scientist makes guesses about which algorithms will best separate the correct answers from the wrong ones. The algorithms are pitted against each other, with the better performing ones moving to the next round of training.
A machine learning algorithm is an algorithm that can assess its own performance and suggest improvements. Training happens in stages, the algorithm is trained, it suggests an improvement, the improvement is applied, and the model is retrained. Training generally ends when the suggested improvements no longer result in better models. However, there are hundreds of such algorithms and choosing the best one is time-consuming. So we create algorithms that train these algorithms, and compete them against each other for us. This cycle has no end, there are algorithms that create algorithms that create algorithms with as many layers as the available computing power can handle. The data scientist knows what the top level target is, and a lot about the top level architecture that encourages the parameters and methods below it to get in line. However, the inner workings of the system itself is a complete black box. It is notoriously difficult to explain in any human way why one image may be classified as containing a bear and another not. Likewise, these systems tell us nothing about how human cognition actually works beyond the simple, unprovable, hypothesis that the winning algorithm might be similar to what the human brain actually uses.
AI is nothing more than a hyper complex system where we throw wet pasta at a wall and iterate on the ones that stick. Over time, we may generate very sticky pasta, but at not point do we ever discover why pasta is sticky or question why it is even desirable that the pasta be sticky.
Turing’s hypothesis, that the perception of an object can replace the existence of an object, sits at the heart of all of this. At not point in the generation of AI is a philosophical definition of its target necessary; in fact, the opposite is true, experiments show it is better to know nothing. The first version of Alpha Go, the algorithm that first beat human masters at the board game Go, had real human games of Go included in its training dataset. The second version, Alpha Zero, only used games that it itself generated through self play. Alpha Zero became the better Go player, demonstrating that trying to insert current human understanding of such a game is actually detrimental to its performance. Or at least that’s the argument that I keep hearing.
Go is a combinatorial board game, meaning that it can be fully modelled mathematically. Anyone who studies games can be easily persuaded that winning at Go is more mathematical than social. We don’t need the imitation game to create a target for go because it already has one built into its rules. There is no ambiguity about what it means to win at go, we don’t even need a physical board to play the game. Go is not an image of its social norms, the mythology surrounding the game is itself the image; so of course the AI will do better when that image is removed, as training on the singular variable we care about will always be better than a simulacrum of that variable. This is why Alpha Zero is not, in my opinion, a good example of why computers will eventually be better at everything than humans. Yet that doesn’t stop people from believing that winning at Go is a natural step in the computer’s evolution towards personhood5.
AI Safety
The above talk by Robert Miles is about AI safety and gives a great overview of the dangers of AI. I highly recommend watching it through before continuing. However, I will do my best to summarize the parts I am particularly interested in.
Miles talks about creating an AI for a boat racing game with a problematic win condition. Racing is different from Go because there is no natural midpoint between winning and not winning. Go has a score system, and whoever has a higher score wins. So we can train an AI to maximize its score without really worrying about what the other players are doing. Naturally, the researchers working on this boat race also choose to train their AI on score, assuming that high scores correlate with winning the race. What the researchers got; however, was an AI that crashed the boat into everything it could before picking up infinitely re-spawning repair tokens that gave it a few points. The AI then drove the boat in circles indefinitely, producing infinite score, but never completing the race. A target that seemed close to the desired goal produced an AI that acted wholly separate from it. Miles makes it clear that this is not an isolated incident; similar bizarre behaviour has been reproduced in many independent studies.
Miles then goes on to explain how this problem is actually a lot worse than that. He uses the example of a robot that makes tea. Such a robot only values what it has been explicitly told to value. So it would likely destroy the priceless Ming vase situated between it and the tea pot because going around the vase would slow it down. If we created a target that valued the vase, the robot would likely destroy the painting beside the vase:
When you are making decisions in the real world, you are always making trade-offs, you are always taking various things that you value and deciding how much of one you are willing to trade for how much of another… An agent like this which only cares about a limited subset of the variables in the system will be willing to trade off arbitrarily large amounts of any of the variables that aren’t a part of its goal for arbitrarily tiny increases in any of the things which are in its goal. …. If you manage to come up with a really good objective function that covers the top twenty things that humans value, the twenty-first thing that humans value is probably gone forever.
Robert Miles
The primary difference between a game like Go and a racing simulation is that Go is a small game in terms of rules. It is possible to accurately model the entire game, along with its goals, in a limited amount of code. At no time is it possible for the politics in India to ever have any impact on a single game of Go. Making tea is not that. Even simple things like ‘the weather’ can blow on the tea making it colder; anything that could interact with the tea needs to be included in its model, yet we cannot make an AI that accurately models the entire universe; we will only ever get an image. A painting is not the same as the thing that is being painted, and no matter how masterful the craftsmanship is, there will always be a separation between an object and its image. Likewise, no matter how powerful a machine learning algorithm is, it can never learn to value that separation. Its theoretical groundwork pretends like it doesn’t exist, and, as Miles Points out, anything that it doesn’t value is just fuel to be traded for the things it does care about. But Turing wasn’t wrong in one key area. Many human things are perception first, and aren’t grounded in physical reality. Yet that only makes the job of mimicking it even harder because we are now modelling an image of an image and the separation only grows.
The desire to reduce human complexity to physical and tangible objects precedes Turing. The idea that an image can replace an object is a religious ideal that has no beginning. In fact, we don’t need to dig into modern computer science to find out what the consequences of machine learning systems are. We already have target values that we use to optimize our societies, and we already have difficulty remembering that these targets are not themselves the things they are supposed to represent. The learning has been going on since before economics itself, and the metric we use today already has a name:
Money.
Money
Chapter four of Adam Smith’s book ‘Wealth of Nations’ acts as most people’s starting point when understanding money. Smith describes money as being a practical necessity. Before money was invented, societies bartered goods back and forth. A butcher has more meat than he needs, and he would like some bread from the baker; naturally they would exchange his meat for the baker’s bread. However, maybe the baker would like the meat, but the butcher doesn’t need bread. How can they exchange goods? In Smith’s telling, the answer is currency. With a separate means of trade, which may begin with things like cattle but inevitably end with gold in all “rich and commercial” nations, a baker can sell bread to someone else and use the money to buy meat from a butcher. Thus, money becomes a practical necessity to facilitate trade; a catalyst that makes other economic activity possible. However, this metaphor is misleading in two crucial ways.
Firstly, these pre-money barter economies never really existed, or so Frederick Kaufman argues in his book ‘The
Money Plot’. For as far back as archaeological evidence will allow us to see, humans have been trading beads, or
other trinkets like eggshells, as currency. Instead, Kaufman provides a different creation narrative for money. In
his telling, money has always been a form of insurance, an object that protects the bearer from future harm
6. Under a modern understanding of money, this includes the classical interpretation as we could use that
money to buy an object we do not yet know we need; however, this can
also take other religious or cultural forms that aren’t understandable to modern eyes. Money can be used to buy
favour from a God, protect against charms and hexes, be used to purchase comfort for a loved one in the afterlife,
or guarantee the ongoing existence of a family line through marriage: all of these according to the values and
beliefs of the society doing the exchange. Kaufman argues that societies have always interacted with their cultural
values through the exchange of currency; even if modern economists might not recognize these objects as money.
Kaufman argues that societies have always interacted with their cultural values through the exchange of currency; even if modern economists might not recognize these objects as money.
Secondly, this tale denies an essential nature of money. If I sell eggs for currency, then the currency I receive is just a signifier for an incomplete transaction. It is only after I use that money to buy something else that the transaction is complete. In this world, money is just an object whose presence is necessary for other more important transactions to occur. This metaphor vastly underestimates the compounding aspect of money and makes it seem like managing money is a simple act of balancing inputs and outputs. As long as the money I receive from my trade is greater than the money I spend, then I will have the cash to grow my material possessions and I will become wealthy. While not necessarily wrong, this view is naive.
Imagine a game with two boxes. One box is made of a clear material and obviously contains one million dollars. The second box is made of an opaque material, and the caretaker has informed us it has an equal chance of containing ten million dollars or nothing. I am allowed to open one box and keep whatever is inside. Which should I open?
The correct answer changes depending on my life situation. For most of us, one million dollars is a life changing amount of money. It is more than enough money to pay off debts, buy a nice house, upgrade skills, or spend several years looking for high-paying and satisfying work. Ten million dollars, on the other hand, is also this, but leaves an additional nine million dollars of unspent money. So from the perspective of barter, the answer here is obvious; why would someone turn down a life changing amount of money for a chance at a life changing amount of money? Any sane person would go for the sure thing and take the million.
Of course, the above answer is wrong if we think through the problem mathematically. The probability of getting ten million dollars if fifty-fifty. If I play the game twice, win once and lose once, I will have gained ten million dollars, eight million more than I would have gained had I gone for the sure thing twice. Sure, we could lose out on a million dollars, but every time we win we gain enough to cover ten different loses. Any sane person would go for the better odds.
The difference between these situations is easier to see if we change the rules of the game a tiny bit. Instead of money, the clear box now contains nothing, and the opaque box contains either nine million dollars or one million in debt. Which do you open? The mathematics of the situation hasn’t changed, it is still better to take the gamble. However, the consequences of losing that gamble are much direr for people who don’t have a life changing amount of money to throw away. A million dollars of debt is a crippling amount, and enough to either ruin or drive to suicide, most who incur it. Yet if you can handle losing, the gains still vastly outweigh the risks. Even a single win is more than enough to pay for multiple loses. On average, the person capable of taking such a risk will gain money faster than those who cannot.
There is a myth that those with money are better at handling finances than someone who doesn’t have it; this is false. The truth is, the more money you have, the fewer variables you need to consider while managing it. To someone living in poverty, every financial decision is a matter of life and death. Sure, they could invest ten dollars and gamble on making a hundred later, or they can buy food and not starve to death. A person with money can afford to leave their job and search for a better one, a luxury a person without money cannot afford. A person with money can risk eviction and negotiate with their landlord for better rent, a person without money cannot. A person with money can risk exploring a new business opportunity, a person without money cannot. Money allows a person to take bigger and more frequent risks. So long as the rewards outweigh the costs, higher risk gambles increase one’s ability to make more money.
There are two mathematical rules that govern risk at this level. The first is the ‘expected value’ formula. It is calculated by multiplying our expected return by the associated risk factor. In the earlier example, the expected value of the clear box is zero, while the expected value of the opaque box is 4 million dollars. The second concept is the ‘law of large numbers’ which states that the more we simulate a random outcome, the closer the actual result will be to its expected value. If I play the game only once I may lose a million dollars, if I play it three more times, I very well could lose every single one of those. However, the probability of getting a winning streak is the same as getting a losing streak and so if I play it enough times then the wins and the loses will balance out, and I will be earning, on average, the expected value every time the game cycles. Unlike the poor person constantly worried about material concerns, the only thing a rich person needs to understand is the expected value of their risks. To the wealthy, money is nothing more than a game of optimizing expected value, and to win they only need accurate information and control over the associated payouts and risks; it is a game of knowledge and power, not inputs and outputs.
To the wealthy, money is nothing more than a game of optimizing expected value, and to win they only need accurate information and control over the associated payouts and risks; it is a game of knowledge and power, not inputs and outputs.
This is why I believe capitalism works so well inside liberal systems. To a capitalist, the only thing worth calculating is risk. Stable Liberal systems, especially globalized ones, make it much easier to calculate long-term risk. If two countries operate in similar ways with similar values, then a profitable gamble in one will also be a profitable gamble in another. Someone else can be paid to manage regional laws, workers and their relationship with the companies, and even the governments trying to regulate these transactions. Everything, from the business to the product, is just a variable, and as long as they remain stable for long enough, risk can be calculated and expected value can be known. So long as expected value is positive, the money will keep increasing so long as we keep gambling.
Yet once the suits at the top of the business start outsourcing everything to other people, and contribute only by pressuring them to generate profitable gambles, the entire structure of our economy begins to look like a machine learning algorithm. The suit is the Data Scientist who defines the target and also the top level algorithms that encourage the layers below it to get in line. So of course, if the top level target is only an image of what the company’s values, then it is only a matter of time before the company as a whole optimizes away something they may have cared about.
The Death of Jupiter Station
So who is at fault when a space station dies? I mentioned in the introduction that a religious organization shut the meta-verse down, but this is a red herring. Religion is just another variable that can be optimized on, and these people may have done the act, but they wouldn’t have been able to do so without powerful help. The station would be primarily inhabited by three different groups of people, each with their own reasons why they would want to see it either destroyed or preserved.
The first group is those who had no choice but to be there. These people may be slaves, indentured servants, or victims of chance who can no longer leave. These people may have plenty of reasons to hate the place, but beyond an act of desperate suicide, none should want to see it destroyed, as doing so would also end their lives. However, as these people are on average less educated, it would be easier for someone more powerful to convince them destruction is not destruction and their rage could easily be converted into a lever for someone else to pull. They may have been involved, but they are not to blame for the stations’ downfall.
The second group are those who choose to be there. These people do have the means to save up and go wherever they want to be, and for one reason or another they choose to orbit Jupiter. Unlike the first group, these people have no reason whatsoever to want the station destroyed, as it is already what they want it to be. The station is their life and its destruction would be their destruction as well, and they know this. Their higher levels of education also mean that their desires would be harder, but not impossible, to use as a tool against the station itself. These people are also not to blame for the stations’ destruction, and many likely did whatever they could to stop it.
The third and final group are those who could be anywhere. They have the means and the power to transform wherever they are into whatever they want it to be. Because of this, they have no attachment to any place in particular. They might like the lifestyle of the station, but they could easily reproduce it on any other station or colony in the solar system. The stations’ loss would hurt, but they can afford that loss because they have the means to build their life somewhere, anywhere, else. These are the people most at fault, because these are the only people who could be plausibly tempted by a box containing the stations destruction. However, even these people aren’t completely at fault.
The true cause lies in the station’s purpose. It is a resource station, it wasn’t built because humans choose to be there, it was built because it provided access to the water on Europa, the geothermal energy on Io, or even the gases surrounding Jupiter itself. It exists to provide more variables that can be transformed into money, or capital, or political power, or whatever else the powerful value. The reason it was destroyed is that the Devil whispered a lie into the ear of its most powerful inhabitant, “If you take this gamble, the expected value for you will be positive.” The suit then agreed to the game, and immediately the devil used the power of that suit’s cooperation to pay preachers, influence governments, discredit education, tell lies, and push its plan to fruition. Did the suit know the station would be destroyed? Maybe or maybe not; the question is irrelevant, as the suit’s only job is to pressure those below him to make money. Did the suit lose a million or gain ten million from the station’s destruction? Also, a meaningless question, as money is a metaphor for value, and we can never know if the suit or the devil valued the station. Is the suit even at fault? Well, that question is also meaningless. If the suit wasn’t the type of person to gamble the station’s existence away, then they wouldn’t have been the suit. The Devil would have pressured them to leave and replaced them with someone else who would have made the gamble. All we can know is that the station itself was just another variable that the devil set to an extreme value to increase another number slightly.
The reason it was destroyed is that the Devil whispered a lie into the ear of its most powerful inhabitant. “If you take this gamble, the expected value for you will be positive.”
As silly as it sounds, the station was destroyed by a super-intelligent AI; Adam Smith’s “invisible hand” made real. However, instead of running on computers, the internet, or whatever “cyberspace” is, this AI runs on economics, and it is just as dangerous as Robert Miles warns us it could be. Its goal is to make money, and everything that is not explicitly money is just a resource to be converted into money: including space stations. The station’s destruction is just a natural consequence of that imperative.
However, there is one final secret I wish to reveal. If the political system that enables the stability necessary for growth becomes a variable to be modified, then the information and assumptions necessary to calculate risk degrade. The result is model collapse; a researched7 phenomenon that happens when the output of an AI system is used to train the next generation of AI. These inputs only reinforce the AI’s hard-coded assumption that what it believes is real actually is real, and its connection to reality degrades. It is what happens when the interrogator in the imitation game is itself replaced with a computer, and we end up trying to convince a computer that a computer is not a computer. Without humans to create images of, we end up creating an image of an image of a human which is nothing more than a hallucination, and the output of such a model becomes garbage with no connection to reality.
So just as the law of large numbers guarantees a positive expected value will create infinite wealth, so too, a negative expected value guarantees bankruptcy. When the devil errors in telling the suit that a gamble is profitable, Smith’s invisible hand will give its final present to itself: its own destruction. I lied when I said the people with means managed to escape Jupiter Station; Jupiter Station is just a metaphor. Nobody did. Nobody does. There is nowhere else to go.
-
I’m using the definition of game I developed in this blog post. ↩
-
Creator of the mathematics of ‘computable numbers’, code breaker instrumental in breaking Nazi encryption during World War 2, and one of the founding fathers of modern computing ↩
-
Turing, Alan. “Computing Machinery and Intelligence”. In The New Media Reader (pp 50-64) online here. ↩
-
Google has been doing this with captchas for years. To decide if someone is human, they show them a bunch of pictures and ask them if they contain traffic signs. Some of these pictures they already know the answer to, others they don’t. If you answered similarly to other humans on the known images you will be declared a human, the rest of the pictures are your unpaid contribution to their dataset. ↩
-
I’ve been working on this blog post for a really long time. I started before ChatGPT blew up the internet. I will admit it is a different beast than Alpha Zero, but I’m still not convinced it is a good argument towards the inevitability of superior AI. The argument below is not about ChatGPT, but it could be with some additional detail. ↩
-
Kaufman, Frederick. “The Money Plot”. pp 9 available here ↩
-
Shumailov, Ilia et al. “The Curse of Recursion: Training on Generated Data Makes Models Forget” online here ↩